Mit der Geostatistical Analyst-Lizenz verfügbar.
Generally speaking, things that are closer together tend to be more alike than things that are farther apart. This is a fundamental geographic principle. Suppose you are a town planner and need to build a scenic park in your town. You have several candidate sites, and you may want to model the viewsheds at each location. This will require a more detailed elevation surface dataset for your study area. Suppose you have preexisting elevation data for 1,000 locations throughout the town. You can use this to build a new elevation surface.
When trying to build the elevation surface, you can assume that the sample values closest to the prediction location will be similar. But how many sample locations should you consider? Should all of the sample values be considered equally? As you move farther away from the prediction location, the influence of the points will decrease. Considering a point too far away may actually be detrimental because the point may be located in an area that is dramatically different from the prediction location.
One solution is to consider enough points to give a good prediction, but few enough points to be practical. The number will vary with the amount and distribution of the sample points and the character of the surface. If the elevation samples are relatively evenly distributed and the surface characteristics do not change significantly across your landscape, you can predict surface values from nearby points with reasonable accuracy. To account for the distance relationship, the values of closer points are usually weighted more heavily than those farther away. This principle is common to all the interpolation methods offered in Geostatistical Analyst (except for global polynomial interpolation, which assigns equal weights to all points).
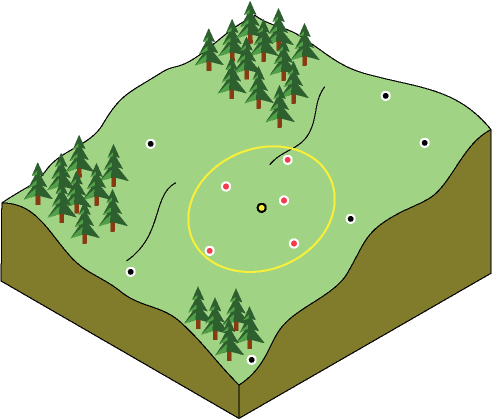