Label | Explanation | Data Type |
Input Space Time Cube
| The space-time cube containing the variable to be analyzed. Space-time cubes have a .nc file extension and are created using various tools in the Space Time Pattern Mining toolbox. | File |
Analysis Variable
| The numeric variable in the netCDF file, changing over time, that will be used to distinguish one cluster from another. | String |
Output Features
| The new output feature class containing all locations in the space-time cube and a field indicating cluster membership. This feature class will be a two-dimensional representation of the clusters in your data. | Feature Class |
Characteristic of Interest
| Specifies the characteristic of the time series that will be used to determine which locations should be clustered together.
| String |
Number of Clusters
(Optional) | The number of clusters to create. When left empty, the tool will evaluate the optimal number of clusters using a pseudo-F statistic. The optimal number of clusters will be reported in the messages window. | Long |
Output Table for Charts
(Optional) | If specified, this table contains the representative time series for each cluster based on both the average for each time series cluster and the medoid time series. Charts created from this table can be accessed in the Standalone Tables section. | Table |
Time Series Characteristics to Ignore
(Optional) | Specifies characteristics that will be ignored when determining the similarity between two time series. If both characteristics are ignored, two time series will be considered similar if the durations of the periods are similar, even if they start at different times and have different values within the periods.
| String |
Enable Time Series Pop-ups
(Optional) | Specifies whether time series charts will be created in the pop-ups of each output feature showing the time series of the feature and the average time series of all features in the same cluster as the feature.
| Boolean |
Summary
Partitions a collection of time series, stored in a space-time cube, based on the similarity of time series characteristics. Time series can be clustered based on three criteria: having similar values across time, tending to increase and decrease at the same time, and having similar repeating patterns. The output of this tool is a 2D map displaying each location in the cube symbolized by cluster membership and messages. The output also includes charts containing information about the representative time series signature for each cluster.
Illustration
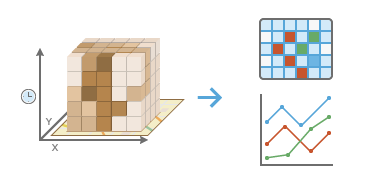
Usage
This tool accepts netCDF files created by various tools in the Space Time Pattern Mining toolbox.
This tool compares the time series at each location to all other locations in the Input Space Time Cube, and time series are clustered together based on their similarity. The Characteristic of Interest parameter is used to define what it means for two time series to be similar, and you can define similarity based on one of the following characteristics:
- Value—Time series are similar if they have approximately equal values of the Analysis Variable across time. For example, a time series with values (1, 0, 1, 0, 1) is more similar to a time series with values (1, 1, 1, 1, 1) than it is to a time series with values (10, 0, 10, 0, 10) because the values are more similar.
- Profile (Correlation)—Time series are similar if their values tend to increase and decrease at the same times and are approximately proportional (in other words, they are correlated across time). For example, a time series with values (1, 0, 1, 0, 1) is more similar to a time series with values (10, 0, 10, 0, 10) than it is to a time series with values (1, 1, 1, 1, 1) because the values increase and decrease at the same time and stay in a consistent proportion.
- Profile (Fourier)—Time series are similar if they have similar smooth, periodic patterns in their values across time. These periods are sometimes called cycles or seasons, and they represent durations of a pattern that then repeats in a new period. For example, businesses may see periodic repeating patterns in their total sales each week, with the period starting on Monday and ending on Sunday. Optionally, you can choose to ignore certain characteristics of these patterns with the Time Series Characteristics to Ignore parameter. The repeating patterns are detected using functional data analysis with a Fourier family. For this option to be most effective, the time series of your input space-time cube should cover the entire duration of at least one period. For example, temperature has a yearly period driven by weather seasons, but if all of the data was collected within several months of a single year, this option may not detect the yearly period.
Using the definition of similarity, the locations of the space-time cube are clustered using one of several clustering algorithms to produce the final clusters returned by the tool. See How Time Series Clustering works for more information about these clustering algorithms.
The Output Features will be added to the Contents pane with rendering based on the CLUSTER_ID field and indicate which cluster each location fell into. If you specify three clusters, for example, each record will contain a value of 1, 2, or 3 for the CLUSTER_ID field. The CENTER_REP field identifies the time series medoid of each cluster and contains a value of 1 for the medoid time series of each cluster and a 0 for all other features.
-
This tool creates messages and optional charts to help you understand the characteristics of the identified clusters. You can access the messages by hovering over the progress bar, clicking the pop-out button, or expanding the messages section in the Geoprocessing pane. You can also access the messages for a previous run of the Time Series Clustering tool using geoprocessing history. If you specify an Output Table for Charts, charts will be created for the output table that display the average time series for each cluster and the medoid of the time series in each cluster. These charts can be accessed in the Contents pane under the table created in the Standalone Tables section. For more information about the output messages and charts, see How Time Series Clustering works.
-
Sometimes you know the number of clusters most appropriate for your data. If you don't, you may need to experiment with different numbers of clusters, noting which values provide the best differentiation between clusters. If you leave the Number of Clusters parameter empty, the tool will evaluate the optimal number of clusters using a pseudo-F statistic and report the optimal number of clusters as a geoprocessing message. The larger the pseudo-F statistic, the more distinct each cluster is from the other clusters. The optimal number of clusters will not be larger than 10, and computing the optimal number of clusters takes most of the execution time of the tool. It is recommended that you provide a number of clusters if you know an appropriate value or if the execution time of the tool is too long.
To calculate the optimal number of clusters, the tool will try between 2 and 10 clusters. For each of these 9 possible number of clusters, the tool will cluster 10 times using random starting seeds (except when Profile (Correlation) is used with more than 10,000 locations in the space-time cube, in which case each number of neighbors is repeated 20 times). This produces 90 (or 180) possible clustering results (10 or 20 for each of the 9 possible number of clusters), and the one with the largest pseudo-F statistic is chosen for the final number of clusters used in the tool. The largest pseudo-F statistic for each of the 9 possible number of clusters is printed as a table in the geoprocessing messages.
Note:
A pseudo-F statistic of infinity means that all time series in the same cluster are perfectly similar to each other.
-
The cluster ID assigned to a location may change from one run to the next because the algorithm randomly selects initial seeds to begin growing clusters. For example, suppose you partition locations into two clusters based on annual population growth. The first time you run the analysis you may see the high growth locations labeled as cluster 2 and the low growth locations labeled as cluster 1; the second time you run the same analysis, the high growth locations may be labeled as cluster 1. You may also see that some of the average or middle growth locations switch cluster membership from one run to another. This is due to a random component in the clustering algorithm. If the clustering results change significantly by rerunning the tool with the same parameters, consider changing the value of the Number of Clusters parameter.
Parameters
arcpy.stpm.TimeSeriesClustering(in_cube, analysis_variable, output_features, characteristic_of_interest, {cluster_count}, {output_table_for_charts}, {shape_characteristic_to_ignore}, {enable_time_series_popups})
Name | Explanation | Data Type |
in_cube | The space-time cube containing the variable to be analyzed. Space-time cubes have a .nc file extension and are created using various tools in the Space Time Pattern Mining toolbox. | File |
analysis_variable | The numeric variable in the netCDF file, changing over time, that will be used to distinguish one cluster from another. | String |
output_features | The new output feature class containing all locations in the space-time cube and a field indicating cluster membership. This feature class will be a two-dimensional representation of the clusters in your data. | Feature Class |
characteristic_of_interest | Specifies the characteristic of the time series that will be used to determine which locations should be clustered together.
| String |
cluster_count (Optional) | The number of clusters to create. When left empty, the tool will evaluate the optimal number of clusters using a pseudo-F statistic. The optimal number of clusters will be reported in the messages window. | Long |
output_table_for_charts (Optional) | If specified, this table contains the representative time series for each cluster based on both the average for each time series cluster and the medoid time series. Charts created from this table can be accessed in the Standalone Tables section. | Table |
shape_characteristic_to_ignore [shape_characteristic_to_ignore,...] (Optional) | Specifies characteristics that will be ignored when determining the similarity between two time series.
If both characteristics are ignored, two time series will be considered similar if the durations of the periods are similar, even if they start at different times and have different values within the periods. | String |
enable_time_series_popups (Optional) | Specifies whether time series charts will be created in the pop-ups of each output feature showing the time series of the feature and the average time series of all features in the same cluster as the feature. Time series pop-ups are not supported for shapefile outputs.
| Boolean |
Code sample
The following Python script demonstrates how to use the TimeSeriesClustering function.
import arcpy
arcpy.env.workspace = r"C:\Analysis"
# Value
arcpy.stpm.TimeSeriesClustering(r"Temperature.nc",
"Air_NONE_ZEROS", r"Analysis.gdb\Temp_Value_3Clusts",
"VALUE", 3, "Temp_Value_3Clusts_Chart", None, "CREATE_POPUP")
# Profile - correlation
arcpy.stpm.TimeSeriesClustering(r"Temperature.nc", "Air_NONE_ZEROS",
r"Analysis.gdb\Temp_Profile_3Clusts", "PROFILE", 3,
r"Temp_Profile_3Clusts_Chart", None, "CREATE_POPUP")
# Profile - Fourier
arcpy.stpm.TimeSeriesClustering(r"Temperature.nc",
"Air_NONE_ZEROS", r"Analysis.gdb\Temp_Fourier_3Clusts",
"PROFILE_FOURIER", 3, r"Temp_Fourier_3Clusts_Chart",
"TIME_LAG", "CREATE_POPUP")
The following Python script demonstrates how to use the TimeSeriesClustering function to cluster similar store locations:
# Create clusters of store locations with similar sales volumes over time.
# Import system modules.
import arcpy
# Set overwriteOutput property to overwrite existing output, by default.
arcpy.env.overwriteOutput = True
# Set workspace...
workspace = r"C:\Analysis"
arcpy.env.workspace = workspace
# Create 3 clusters of location with similar extent of fluctuation in temperature.
arcpy.stpm.TimeSeriesClustering(r"Temperature.nc", "Air_NONE_ZEROS",
r"Analysis.gdb\Temperature_TSC",
"PROFILE_FOURIER", 3, "Temp_Chart", None,
"CREATE_POPUP")
# Create a feature class containing all the bins in the input space time cube.
arcpy.stpm.VisualizeSpaceTimeCube3D(r"Temperature.nc", "Air_NONE_ZEROS", "VALUE",
r"Temp_Bins.shp")
# Make the bins as a feature layer.
arcpy.management.MakeFeatureLayer("Temp_Bins.shp", "Temp_Bins_Temp_Layer")
# Join the clustering results to the bins so each bin now has a cluster ID.
arcpy.management.AddJoin("Temp_Bins_Temp_Layer", "Location",
r"Analysis.gdb\Temperature_TSC", "Location", "KEEP_ALL")
# Summarize the bins using Summary Statistics with Cluster ID as a case field
# to get the minimum, maximum, and average temperature for each cluster.
arcpy.analysis.Statistics("Temp_Bins_Temp_Layer", "Temp_Bins_Statistics.shp",
"Temp_Bins.VALUE MEAN;Temp_Bins.VALUE MAX;Temp_Bins.VALUE MIN",
"Temperature_TSC.CLUSTER_ID")
Environments
Special cases
- Random number generator
The Random Generator Type used is always Mersenne Twister.
Licensing information
- Basic: Yes
- Standard: Yes
- Advanced: Yes