Disponible avec une licence Geostatistical Analyst.
There are two types of directional components that can affect the predictions in your output surface: global trends and directional influences on the semivariogram/covariance (known as anisotropy). A global trend is an overriding process that affects all measurements in a deterministic manner. The global trend can be represented by a mathematical formula (for example, a polynomial) and removed from the analysis of the measured points but added back before predictions are made. This process is referred to as detrending.
Trend
An example of a global trend can be seen in the effects of the prevailing winds on a smokestack at a factory. In the image, the higher concentrations of pollution are depicted in the warm colors (reds and yellows) and the lower concentrations in the cool colors (greens and blues). Notice that the values of the pollutant change more slowly in the east–west direction than in the north–south direction. This is because east–west is aligned with the wind while north–south is perpendicular to the wind.
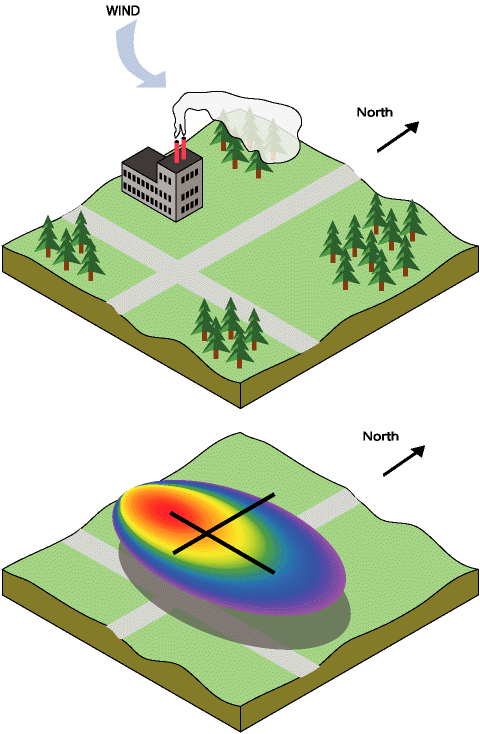
The shape of the semivariogram/covariance curve may also vary with direction (anisotropy) after the global trend is removed or if no trend exists. Anisotropy differs from the global trend discussed above because the global trend can be described by a physical process (the prevailing winds) and modeled by a mathematical formula. The cause of the anisotropy (directional influence) in the semivariogram is not usually known, so it is modeled as a random error. Even without knowing the cause, anisotropic influences can be quantified and accounted for.
Anisotropy
Anisotropy is usually not a deterministic process that can be described by a single mathematical formula. It does not have a single source or influence that predictably affects all measured points. Anisotropy is a characteristic of a random process that shows higher autocorrelation in one direction than another. The following image shows conceptually how the process might look. Once again, the higher concentrations of pollution are depicted in the warm colors (reds and yellows) and the lower concentrations in the cool colors (greens and blues). The random process shows undulations that are shorter in one direction than another. These undulations could be the result of some unknown or unmeasurable physical process but are modeled as a random process with directional autocorrelation.
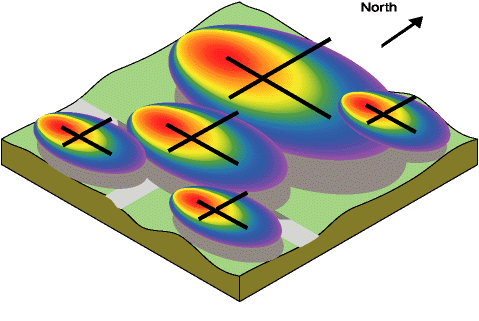
When you use a kriging method and choose to account for anisotropy, the empirical semivariogram will show a different spatial relationship for each direction. In this example, the shape of the semivariogram will increases more rapidly in the north–south direction before leveling out (this is the direction where the concentrations change more rapidly). The empirical semivariogram points will be fitted using semivariogram models that change shape depending on their direction, and the kriging weights will adjusted accordingly.
Deterministic methods can also account for anisotropy, even though they do not provide an empirical measure of its strength or direction. To account for anisotropy when using these methods, you must judge the level of anisotropy based on an exploratory spatial data analysis and your knowledge of the phenomenon. Interpolation weights will be adjusted based on how you set up the search neighborhood ellipse (data points located along the major semiaxis will receive higher weights than those located along the minor semiaxis, for similar distances from the prediction location).
Vous avez un commentaire à formuler concernant cette rubrique ?