描述
使用 Leo Breiman 随机森林算法(一种监督式机器学习方法)的改编版本创建模型并生成预测。可以针对分类变量(分类)和连续变量(回归)执行预测。解释变量可以采取用于计算邻域分析值的训练要素、栅格数据集和距离要素的属性表中字段的形式,以用作附加变量。除了基于训练数据对模型性能进行验证之外,还可以对要素或预测栅格进行预测。
插图
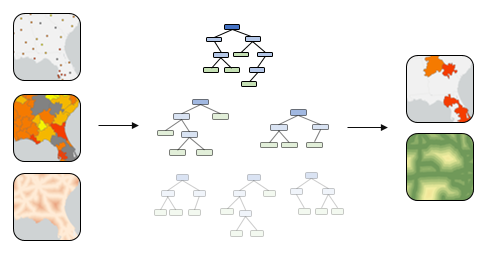
使用方法
该工具将创建数百棵树(称为决策树集成),从而创建可用于预测的模型。将使用原始(训练)数据的随机生成部分创建每棵决策树。每棵树可以生成自己的预测并对结果进行投票。森林模型将考虑所有决策树的投票,以预测或分类未知样本的结果。此功能非常重要,因为单棵树可能存在过度拟合模型的问题;但森林中的多棵树进行预测可解决与单棵树相关的过度拟合问题。
可以在三种不同的操作模式下使用此工具。探索不同的解释变量和工具设置时,可以使用训练选项来评估不同模型的性能。找到合适的模型后,可以使用预测至要素或预测至栅格选项。这是一个数据驱动工具,在大型数据集上性能最佳。为获得最佳结果,应针对至少数百个要素对该工具进行训练。该工具不适用于非常小的数据集。
输入训练要素可以是点或面。而且,此工具不能用于处理多点数据。
要使用栅格作为解释变量或预测至输出预测表面,需要 Spatial Analyst 许可。
该工具会生成各种不同的输出。输出训练要素将包含在创建的模型中使用的所有输入训练要素以及在模型中使用的所有解释变量(包括使用的输入字段、计算的所有距离,以及提取或计算的所有栅格值)。还将包含用于训练模型的所有要素的预测,这对于评估所创建模型的性能非常有用。使用此工具进行预测时,它将生成一个新的要素类(包含输出预测要素)或新的输出预测表面(如果已提供解释栅格)。
如果使用预测至要素选项,则将创建一个新的要素类,其中包含输出预测要素。如果使用预测至栅格选项,则将创建一个新的输出预测表面。
该工具还会创建消息和图表,以帮助您了解所创建模型的性能。可将鼠标悬停在进度条上、单击弹出按钮或展开地理处理窗格中的消息部分来访问消息。还可通过地理处理历史访问之前运行基于森林的分类和预测工具的消息。这些消息包括有关模型特征、袋外误差、变量重要性以及验证诊断的信息。
可以使用输出变量重要性表格参数创建一个表格,用于显示变量重要性的图表以进行评估。消息窗口中还会报告前 20 个变量重要性值。可以在内容窗格中的图层下方直接访问该图表。
解释变量可以来自字段、可通过距离要素进行计算得出或者从栅格中提取得出。可以使用这些解释变量类型的任意组合,但至少要使用一种类型。使用的解释变量(来自字段、距离要素或栅格)应该包含各种值。如果解释变量为分类变量,应选中分类复选框(将自动选中字符串类型的变量)。尽管较小的类别数量可改善模型性能,但分类解释变量限于 60 个唯一值。对于给定的数据大小,变量所包含的类别越多,则其影响模型的可能性越大,从而导致预测结果的有效性越低。
距离要素用于自动创建解释变量,这些解释变量表示给定要素与输入训练要素的距离。将计算每个输入解释训练距离要素与最近的输入训练要素的距离。如果输入解释训练距离要素为面要素或线要素,则距离属性将计算为要素对的最近线段之间的距离。但是,面要素和线要素的距离计算方式不同。有关详细信息,请参阅邻近分析工具计算距离的方法。
如果输入训练要素为点要素并且使用解释训练栅格,则工具将向下钻取以提取每个点位置处的解释变量。对于多波段栅格,仅使用第一个波段。
虽然内容窗格中可以存在多个具有相同名称的图层,但该工具无法接受具有相同名称的解释图层,或者无法移除下拉列表中的重复图层名称。要避免此问题,请确保每个图层都具有唯一名称。
如果输入训练要素为面要素,要预测的变量为分类变量,并且独占使用解释训练栅格,则可以选择将面转换为栅格分辨率以进行训练。如果选中此选项,则将在每个栅格像元(其质心落在面范围内)的质心处将面划分为点。然后,将提取每个点位置处的栅格值,然后将其用于训练模型。对于数值变量,将使用双线性采样方法;对于分类变量,将使用最邻近方法。经过转换的面要素的默认像元大小将为输入栅格的最大像元大小。但是,可以使用像元大小环境设置对其进行更改。如果未选中此选项,则将在模型中针对每个面使用一个栅格值。将为每个面指定连续栅格的平均值和分类栅格的众数。
面转换为栅格分辨率(左)或分配平均值(右) 用于每个指定的解释变量的数据必须存在差异。如果您收到错误,指示某个指定的字段或栅格中没有差异,则可以尝试再次运行此工具,将该变量标记为分类变量。如果 95% 的要素对于特定变量具有相同的值,则可将此变量标记为没有差异。
如果类别中的变化不平衡,则可以使用补偿稀疏类别参数。例如,如果某些类别在数据集中出现数百次,而少数类别出现的次数明显较少,则选中此参数将确保每棵树中存在用于创建平衡模型的各个类别。
对解释变量进行匹配时,Prediction 和 Training 字段的类型必须相同(Training 中的双精度字段必须与 Prediction 中的双精度字段相匹配)。
基于森林模型不会进行外推,只能对模型训练所使用的值进行分类或预测。基于高于或低于原始训练数据集范围的解释变量预测值时,模型将预测值位于原始数据集中最高值或最低值的附近。尝试使用超出用于训练模型的解释变量范围的解释变量进行预测时,该工具可能性能不佳。
如果类别存在于训练要素中不存在的预测解释变量中,则该工具将失败。
要将镶嵌数据集用作解释变量,请首先使用创建镶嵌图层工具,然后将图层的完整路径复制到工具中,或使用创建镶嵌图层和创建栅格图层工具来调整镶嵌数据集的处理模板。
树数参数的默认值为 100。增加森林模型中的树数将使模型预测更加精准,但是将增加模型计算的时间。
选中计算不确定性参数时,该工具将计算出要预测的变量中每个预测值周围 90% 的预测区间。当预测类型为仅训练或预测要素时,则两个字段会添加到输出已训练要素或输出预测要素中。这些字段以 _P05 和 _P95 结尾,表示预测区间的上界和下界。对于任何新的观测点,在给定相同解释变量的情况下,您可以 90% 的置信度预测新观测点将位于区间内。使用预测栅格选项时,表示预测区间的上限和下限的两个附加栅格被添加到内容窗格。
此工具支持并行处理预测,默认情况下使用 50% 的可用处理器。处理器数目可以通过使用并行处理因子环境增加或减少。
要了解有关此工具工作原理的详细信息并理解输出消息和图表,请参阅基于森林分类和回归工作原理。
参考:
Breiman, Leo. Out-Of-Bag Estimation. 1996.
Breiman, L. (1996). Bagging predictors. Machine learning, 24(2), 123-140.
Breiman, Leo. "Random Forests". Machine Learning. 45 (1): 5-32. doi:10.1023/A:1010933404324. 2001.
Breiman, L., J.H. Friedman, R.A. Olshen, C.J. Stone. Classification and regression trees. New York: Routledge. Chapter 4. 2017.
Dietterich, T. G. (2000, June). Ensemble methods in machine learning. 选自 International workshop on multiple classifier systems (pp. 1-15)。Springer, Berlin, Heidelberg.
Gini, C. (1912). Variabilità e mutabilità. Memorie di metodologica statistica (Ed. Pizetti E, Salvemini, T) 转载。Rome: Libreria Eredi Virgilio Veschi.
Grömping, U. (2009). Variable importance assessment in regression: linear regression versus random forest. The American Statistician, 63(4), 308-319.
Ho, T. K. (1995, August). Random decision forests. 选自 Document analysis and recognition, 1995., proceedings of the third international conference on Document Analysis and Recognition.。(Vol. 1, pp. 278-282)。IEEE.
James, G., Witten, D., Hastie, T., & Tibshirani, R. (2013). An introduction to statistical learning (Vol. 112). New York: springer.
LeBlanc, M., & Tibshirani, R. (1996). Combining estimates in regression and classification. Journal of the American Statistical Association, 91(436), 1641-1650.
Loh, W. Y., & Shih, Y. S. (1997). Split selection methods for classification trees. Statistica sinica, 815-840.
Meinshausen, Nicolai. "Quantile regression forests." Journal of Machine Learning Research 7. Jun (2006): 983-999.
Nadeau, C., & Bengio, Y. (2000). Inference for the generalization error. 选自 Advances in neural information processing systems (pp. 307-313)。
Strobl, C., Boulesteix, A. L., Kneib, T., Augustin, T., & Zeileis, A. (2008). Conditional variable importance for random forests. BMC bioinformatics, 9(1), 307.
Zhou, Z. H. (2012). Ensemble methods: foundations and algorithms. CRC press.
语法
Forest(prediction_type, in_features, {variable_predict}, {treat_variable_as_categorical}, {explanatory_variables}, {distance_features}, {explanatory_rasters}, {features_to_predict}, {output_features}, {output_raster}, {explanatory_variable_matching}, {explanatory_distance_matching}, {explanatory_rasters_matching}, {output_trained_features}, {output_importance_table}, {use_raster_values}, {number_of_trees}, {minimum_leaf_size}, {maximum_depth}, {sample_size}, {random_variables}, {percentage_for_training}, {output_classification_table}, {output_validation_table}, {compensate_sparse_categories}, {number_validation_runs}, {calculate_uncertainty})
参数 | 说明 | 数据类型 |
prediction_type | 指定工具的操作模式。可以运行此工具来训练模型,以仅评估性能、预测要素或创建预测表面。
| String |
in_features | 要素类,包含 variable_predict 参数以及字段中的解释训练变量(可选)。 | Feature Layer |
variable_predict (可选) | in_features 参数中的变量,其中包含要用于训练模型的值。该字段包含将用于在未知位置进行预测的变量的已知(训练)值。 | Field |
treat_variable_as_categorical (可选) |
| Boolean |
explanatory_variables [[Variable, Categorical],...] (可选) | 表示解释变量的字段列表,可帮助预测 variable_predict 的值或类别。对于任何表示类或类别(例如土地覆被或存在/不存在)的变量,请使用 treat_variable_as_categorical 参数。对于任何表示类或类别(例如土地覆被或存在/不存在)的变量,请指定变量为 true;如果变量为连续变量,请指定变量为 false。 | Value Table |
distance_features [distance_features,...] (可选) | 通过计算给定要素与 in_features 的距离可自动创建解释变量。将计算每个输入 distance_features 与最近的 in_features 的距离。如果输入 distance_features 为面要素或线要素,则距离属性将计算为要素对的最近线段之间的距离。 | Feature Layer |
explanatory_rasters [[Variable, Categorical],...] (可选) | 将在模型(其值从栅格中提取)中自动创建解释训练变量。对于 in_features 中的每个要素,将在此确切位置处提取栅格像元的值。提取栅格值时,将使用双线性栅格重采样;如果指定栅格为分类栅格,则将使用最邻近分配法。对于任何表示类或类别(例如土地覆被或存在/不存在)的栅格,请指定栅格为 true;如果栅格为连续栅格,请指定栅格为 false。 | Value Table |
features_to_predict (可选) | 表示将进行预测的位置的要素类。此要素类还必须包含作为字段提供的任何解释变量,这些字段对应于训练数据(如果存在)中使用的字段。 | Feature Layer |
output_features (可选) | 用于接收预测结果的输出要素类。 | Feature Class |
output_raster (可选) | 包含预测结果的输出栅格。默认像元大小将为栅格输入的最大像元大小。要设置其他像元大小,请使用像元大小环境设置。 | Raster Dataset |
explanatory_variable_matching [[Prediction, Training],...] (可选) | 根据右侧 in_features 以及左侧 features_to_predict 中其对应字段指定的 explanatory_variables 列表,例如 [["LandCover2000", "LandCover2010"], ["Income", "PerCapitaIncome"]]。 | Value Table |
explanatory_distance_matching [[Prediction, Training],...] (可选) | 针对右侧 in_features 指定的 distance_features 列表。应该针对左侧 features_to_predict 指定对应的要素集。 如果用于训练的要素位于不同的研究区域或时间段,则可以提供更适用于 features_to_predict 的 explanatory_distance_features。 | Value Table |
explanatory_rasters_matching [[Prediction, Training],...] (可选) | 针对右侧 in_features 指定的 explanatory_rasters 列表。应该针对左侧要创建的 features_to_predict 或 output_raster 指定对应的栅格。 如果用于训练的要素位于不同的研究区域或时间段,则可以提供更适用于 features_to_predict 的 explanatory_rasters。 | Value Table |
output_trained_features (可选) | output_trained_features 将包含所有用于训练(包括采样栅格值和距离计算)的解释变量、观察的 variable_to_predict 字段,以及可用于进一步评估训练模型性能的相应预测。 | Feature Class |
output_importance_table (可选) | 如果指定,则表格将包含描述在所创建的模型中使用的每个解释变量(字段、距离要素和栅格)的重要性的信息。 | Table |
use_raster_values (可选) | 如果 in_features 为面要素(variable_predict 为分类变量),并且仅指定了 explanatory_rasters,则训练模型时需要指定面的处理方式。
| Boolean |
number_of_trees (可选) | 要在森林模型中创建的树的数量。增大树数通常将产生更加精确的模型预测,但是将增加模型计算的时间。默认树数为 100。 | Long |
minimum_leaf_size (可选) | 保留叶子(即未进一步进行分割的树上的终端节点)所需的最小观测值数。回归的默认最小值为 5,分类的默认值为 1。对于非常大的数据,增大这些数值将减小工具的运行时间。 | Long |
maximum_depth (可选) | 对树进行的最大分割数。如果使用较大的最大深度,则将创建更多分割,这可能会增大过度拟合模型的可能性。默认值由数据驱动,并且取决于所创建的树数以及所包含的变量数。 | Long |
sample_size (可选) | 指定用于每棵决策树的 in_features 的百分比。默认值为 100% 的数据。将根据指定数据的三分之二随机获取每棵树的样本。 可以使用可用训练数据的随机样本或子集(大约三分之二)来创建森林中的每棵决策树。针对每棵决策树使用较低百分比的输入数据可以提高适用于大型数据集的工具的速度。 | Long |
random_variables (可选) | 指定用于创建每棵决策树的解释变量数。 可以使用指定解释变量的随机子集创建森林中的每棵决策树。增大每棵决策树中使用的变量数将增大过度拟合模型的可能性,尤其是存在一个或多个主导变量时更是如此。常用方法是:如果 variable_predict 为数值,则使用解释变量(组合字段、距离和栅格)总数的平方根;如果 variable_predict 为分类变量,则将解释变量(组合字段、距离和栅格)的总数除以 3。 | Long |
percentage_for_training (可选) | 指定要保留为验证测试数据集的 in_features 的百分比(介于 10% 和 50% 之间)。将在没有此随机数据子集的情况下对模型进行训练,并将这些要素的观测值与预测值进行比较。默认值为 10%。 | Double |
output_classification_table (可选) | 如果指定,则将创建一个混淆矩阵,用于分类汇总所创建模型的性能。除了工具在输出消息中计算的精度和灵敏度测量值之外,此表格还可以用于计算其他诊断。 | Table |
output_validation_table (可选) | 如果指定验证的运行次数大于 2,则此表将为每个模型创建 R2 分布图表。此分布可用于评估模型的稳定性。 | Table |
compensate_sparse_categories (可选) | 如果数据集中存在出现频繁低于其他类别的类别,则选中此参数将确保每棵树中存在各个类别。
| Boolean |
number_validation_runs (可选) | 工具将运行指定迭代次数。可以使用输出验证表参数来显示每次运行的 R2 分布。设置此选项并生成预测后,仅生成了最高 R2 值的模型将用于预测。 | Long |
calculate_uncertainty (可选) | 指定在训练、预测要素或预测栅格时是否计算预测不确定性。
| Boolean |
派生输出
名称 | 说明 | 数据类型 |
output_uncertainty_raster_layers | 选中 calculate_uncertainty 时,该工具将计算出 variable_to_predict 中每个预测值周围 90% 的预测区间。 | 栅格图层 |
代码示例
以下 Python 脚本演示了如何使用 Forest 函数。
import arcpy
arcpy.env.workspace = r"c:\data"
# Forest-based model using only the training method and all data
# comes from a single polygon feature class. The tool excludes 10% of the
# input features from training and uses these values to validate the model.
prediction_type = "TRAIN"
in_features = r"Boston_Vandalism.shp"
variable_predict = "VandCnt"
explanatory_variables = [["Educat", "false"], ["MedAge", "false"],
["HHInc", "false"], ["Pop", "false"]]
output_trained_features = "TrainingFeatures.shp"
number_of_trees = 100
sample_size = 100
percentage_for_training = 10
arcpy.stats.Forest(prediction_type, in_features, variable_predict, None,
explanatory_variables, None, None, None, None, None, None, None, None,
output_trained_features, None, True, number_of_trees, None, None, sample_size,
None, percentage_for_training)
以下 Python 脚本演示了如何使用 Forest 函数以预测至要素。
# Import system modules
import arcpy
# Set property to overwrite existing outputs
arcpy.env.overwriteOutput = True
# Set the work space to a gdb
arcpy.env.workspace = r"C:\Data\BostonCrimeDB.gdb"
# Forest-based model taking advantage of both distance features and
# explanatory rasters. The training and prediction data has been manually
# split so the percentage to exclude parameter was set to 0. A variable importance
# table is created to help assess results and advanced options have been used
# to fine tune the model.
prediction_type = "PREDICT_FEATURES"
in_features = r"Boston_Vandalism_Training"
variable_predict = "Vandalism_Count"
treat_variable_as_categorical = None
explanatory_variables = [["EduClass", "true"], ["MedianAge", "false"],
["HouseholdIncome", "false"], ["TotalPopulation", "false"]]
distance_features = r"Boston_Highways"
explanatory_rasters = r"LandUse true"
features_to_predict = r"Boston_Vandalism_Prediction"
output_features = r"Prediction_Output"
output_raster = None
explanatory_variable_matching = [["EduClass", "EduClass"], ["MedianAge", "MedianAge"],
["HouseholdIncome", "HouseholdIncome"], ["TotalPopulation", "TotalPopulation"]]
explanatory_distance_matching = [["Boston_Highways", "Boston_Highways"]]
explanatory_rasters_matching = [["LandUse", "LandUse"]]
output_trained_features = r"Training_Output"
output_importance_table = r"Variable_Importance"
use_raster_values = True
number_of_trees = 100
minimum_leaf_size = 2
maximum_level = 5
sample_size = 100
random_sample = 3
percentage_for_training = 0
arcpy.stats.Forest(prediction_type, in_features, variable_predict,
treat_variable_as_categorical, explanatory_variables, distance_features,
explanatory_rasters, features_to_predict, output_features, output_raster,
explanatory_variable_matching, explanatory_distance_matching,
explanatory_rasters_matching, output_trained_features, output_importance_table,
use_raster_values, number_of_trees, minimum_leaf_size, maximum_level,
sample_size, random_sample, percentage_for_training)
以下 Python 脚本演示了如何使用 Forest 函数以创建预测表面。
# Import system modules import arcpy
# Set property to overwrite existing outputs arcpy.env.overwriteOutput = True
# Set the work space to a gdb arcpy.env.workspace = r"C:\Data\Landsat.gdb"
# Using a forest-based model to classify a landsat image. The TrainingPolygons feature
# class was created manually and is used to train the model to
# classify the remainder of the landsat image.
prediction_type = "PREDICT_RASTER"
in_features = r"TrainingPolygons"
variable_predict = "LandClassName"
treat_variable_as_categorical = "CATEGORICAL" explanatory_variables = None distance_features = None explanatory_rasters = [["Band1", "false"], ["Band2", "false"], ["Band3", "false"]] features_to_predict = None output_features = None output_raster = r"PredictionSurface"
explanatory_variable_matching = None explanatory_distance_matching = None explanatory_rasters_matching = [["Band1", "Band1"], ["Band2", "Band2"], ["Band3", "Band3"]] output_trained_features = None output_importance_table = None use_raster_values = True number_of_trees = 100 minimum_leaf_size = None maximum_level = None sample_size = 100 random_sample = None percentage_for_training = 10
arcpy.stats.Forest(prediction_type, in_features, variable_predict, treat_variable_as_categorical, explanatory_variables, distance_features, explanatory_rasters, features_to_predict, output_features, output_raster, explanatory_variable_matching, explanatory_distance_matching, explanatory_rasters_matching, output_trained_features, output_importance_table,
use_raster_values, number_of_trees, minimum_leaf_size, maximum_level, sample_size, random_sample, percentage_for_training)
许可信息
- Basic: 受限
- Standard: 受限
- Advanced: 受限