插图
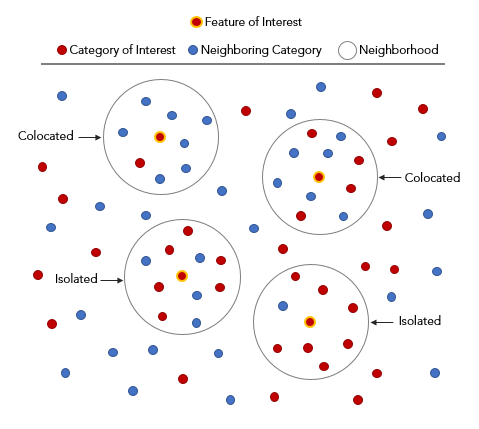
使用方法
该工具仅接受点要素。要分析的类别可以位于同一数据集或两个单独的数据集中。也可以将两个单独的数据集视为类别。例如,对于包含许多类型餐厅的点数据集,只能将其视为类别 RESTAURANTS;对于包含许多类型犯罪的另一个点数据集,只能将其视为类别 CRIMES。
该工具将针对感兴趣类别的每个要素确定,与类别的整体空间分布相比,相邻类别的要素出现在其邻域内的可能性更大还是更小。例如,对于类别 A 的每个要素,生成的局部协同区位商 (LCLQ) 值为 1 时,意味着很可能与预期一样相邻要素为类别 B。LCLQ 值大于 1 时,意味着相邻要素为 B 的可能性更大(与随机相比),而 LCLQ 值小于 1 时,意味着类别 A 的要素的相邻要素为类别 B 点的可能性不大(与随机分布相比)。
注:
此分析的协同区位关系不对称。将类别 A 与类别 B 进行比较时计算的协同区位商值将不同于将类别 B 与类别 A 进行比较时计算的协同区位商值。
此外,如果邻域中存在类别 C,则与只有类别 A 和 B 相比,生成的协同区位商将有所不同。根据要询问的问题,可能需要创建数据子集以仅包含类别 A 和 B。但是,在创建子集时,您将失去有关存在的其他类别的信息。如果您确定某一类别的出现完全不受另一类别出现的影响,则选择并创建数据子集至关重要。
空间关系可以使用距离范围、最近的 K 个相邻要素或空间权重矩阵文件通过邻域类型参数进行定义。
可以使用空间时间窗口来分析数据,方法是指定感兴趣的时间字段、相邻类别的时间字段和时间关系类型参数。使用空间时间窗口,可控制哪些要素包含在所分析的邻域中。在空间和时间上彼此相邻的要素将一起分析,因为所有要素关系都是相对于目标要素的位置和时间戳进行评估的。也可以指定该工具是在目标特征之前还是之后搜索特征,或者可以创建一个时间跨度,在该跨度内该工具将在要分析的目标特征之前和之后搜索特征。
置换检验次数参数用于计算 p 值。选择置换检验次数时,需要兼顾精度和所需增加的处理时间。默认值为 99 次置换检验,但建议增加最终分析结果的置换检验次数。
要计算全局协同区位商,也可以通过为全局关系输出表参数指定路径。该表包含区位商,因此可以分析数据集中所有类别之间的空间关联测量值。从而可以探索数据中的其他关系,因为可能会在全局范围内找到其他高度区位协同的类别。如果您确实找到了其他高度区位协同的类别,则可以通过以下方式扩展分析:通过使用感兴趣的类别再次运行该工具来探索该关系的局部性质;或者如果您认为高度区位协同的类别会在您的结果中引入不必要的偏差,则通过从分析中移除这些类别再次运行该工具。
该工具的输出是一张地图,其中显示的每个感兴趣的输入要素按照其与输入相邻要素是明显区位协同还是相互隔离进行符号化。该工具会向输出要素添加字段,包括计算的局部协同区位商、p 值、用于符号化的 LCLQ 立方图格和 LCLQ 类型。可以指定可选的全局关系输出表,以报告感兴趣字段参数中所有类别和包含相邻类别的字段参数中存在的所有类别之间的全局协同区位商。
此工具支持并行处理,默认情况下使用 50% 的可用处理器。处理器数目可以通过使用并行处理因子环境增加或减少。
语法
arcpy.stats.ColocationAnalysis(input_type, in_features_of_interest, output_features, {field_of_interest}, {time_field_of_interest}, {category_of_interest}, {input_feature_for_comparison}, {field_for_comparison}, {time_field_for_comparison}, {category_for_comparison}, neighborhood_type, {number_of_neighbors}, {distance_band}, {weights_matrix_file}, {temporal_relationship_type}, {time_step_interval}, {number_of_permutations}, {local_weighting_scheme}, {output_table})
参数 | 说明 | 数据类型 |
input_type | 指定 in_features_of_interest 参数值是来自包含指定类别的同一数据集、包含指定类别的不同数据集还是将被视为其自身类别的不同数据集(例如,一个数据集包含表示猎豹的所有点,另一个数据集包含表示瞪羚的所有点)。
| String |
in_features_of_interest | 包含具有代表性类别的点的要素类。 | Feature Layer |
output_features | 该输出要素类包含所有 in_features 参数值并具有表示局部协同区位商分数和 p 值的字段。 | Feature Class |
field_of_interest (可选) | 包含要分析的一个或多个类别的字段。 | Field |
time_field_of_interest (可选) | 具有每个要素的可选时间戳的日期字段,以供使用空间时间窗口分析点。在空间和时间上彼此相邻的要素将被视为相邻要素,并将一起进行分析。 | Field |
category_of_interest (可选) | 分析的基本类别。该工具将针对每个 category_of_interest 值确定,基本类别被 neighboring_category 参数值吸引或与之区位协同的程度。 | String |
input_feature_for_comparison (可选) | 该输入要素类包含具有要比较的类别的点。 | Feature Layer |
field_for_comparison (可选) | 来自 input_feature_for_comparison 参数的字段,包含要比较的类别。 | Field |
time_field_for_comparison (可选) | 具有每个要素的时间戳的日期字段,以供使用空间时间窗分析点。在空间和时间上彼此相邻的要素将被视为相邻要素,并将一起进行分析。 | Field |
category_for_comparison (可选) | 分析的相邻类别。该工具将确定 category_of_interest 参数值被 category_for_comparison 值吸引或与之相互隔离的程度。 | String |
neighborhood_type | 指定要素空间关系的定义方式。
| String |
number_of_neighbors (可选) | 每个要素周围用于测试类别之间的局部关系的相邻要素数。如果未指定任何值,则将使用默认值 8。提供的值必须足够大,才能检测要素之间的关系,但同时要小到足以识别局部模式。 | Long |
distance_band (可选) | 邻域大小是每个要素的恒定或固定距离。此距离内的所有要素都将用于测试类别之间的局部关系。如果未提供任何值,则使用的距离将是每个要素至少具有八个相邻要素的平均距离。 | Linear Unit |
weights_matrix_file (可选) | 包含权重(其定义要素间的空间关系以及可能的时态关系)的文件的路径。 | File |
temporal_relationship_type (可选) | 指定要素时态关系的定义方式。
| String |
time_step_interval (可选) | 表示构成时间窗口的时间单位数的整数和测量单位。 | Time Unit |
number_of_permutations (可选) | 用于创建参考分布的置换检验次数。选择置换检验次数时,需要兼顾精度和所需增加的处理时间。根据偏好选择速度或精度。结果越可靠越精确计算所花费的时间就会越长。
| Long |
local_weighting_scheme (可选) | 指定用于提供空间加权的核类型。核用于定义每个要素与其邻域内其他要素的关联方式。
| String |
output_table (可选) | 包含感兴趣字段参数中所有类别和包含相邻类别的字段参数中所有类别之间的全局协同区位商的表格。此表可帮助您确定要分析的局部类别。 如果将不含类别的数据集用作输入类型参数值,则将计算每个数据集和各个数据集之间的全局协同区位商。 | Table |
代码示例
以下 Python 窗口脚本演示了如何使用 ColocationAnalysis 函数。
import arcpy
arcpy.env.workspace = r"C:\Analysis"
# Two categories from the same categorical field.
# Find the colocation of elementary schools and middle schools
arcpy.stats.ColocationAnalysis("SINGLE_DATASET", r"Colocation.gdb\Schools",
r"Outputs.gdb\School_Colocation", "Facility_Type", None,
"Elementary", None, None, None, "Middle", "K_NEAREST_NEIGHBORS",
8, None, None, "BEFORE", None, 99, "BISQUARE",
r"Outputs.gdb\Global_School_Colocation")
# Categories from different datasets without categories
# Find the colocation of elementary schools and hospitals
arcpy.stats.ColocationAnalysis("DATASETS_WITHOUT_CATEGORIES", r"Colocation.gdb\Schools",
r"Outputs.gdb\Schools_Hospitals", None, None, '',
r"Colocation.gdb\Hospitals", None, None, '', "DISTANCE_BAND",
None, "30 Kilometers", None, "BEFORE", None, 199, "GAUSSIAN",
None)
# Categories from two datasets
# Find the colocation of elementary schools and hospitals
arcpy.stats.ColocationAnalysis("TWO_DATASETS", r"Colocation.gdb\Schools",
r"Outputs.gdb\Elementary_Hospitals", "Facility_Type", None,
"Elementary", r"Colocation.gdb\Hospitals", None, None, '',
"K_NEAREST_NEIGHBORS", 15, None, None, "BEFORE", None, 499,
"NONE", None)
以下独立 Python 脚本演示了如何使用 ColocationAnalysis 函数。
# Analyze the spatial relationship (colocation) between elementary school locations and hospital locations
# Two categories from the same categorical field.
# Find the colocation of elementary schools and middle schools
intype = "SINGLE_DATASET"
infc_interest = r"Colocation.gdb\Schools"
outfc = r"Outputs.gdb\School_Colocation"
field_interest = "Facility_Type"
time_field = ""
cat_interest = "Elementary"
infc_neigh = ""
field_neigh = ""
time_field_neigh = ""
cat_neigh = "Middle"
neighborhood_type = "K_NEAREST_NEIGHBORS"
num_neighbors = 8
dist_band = ""
swm_file = ""
temporal_type = ""
time_step_interval = ""
num_permutation = 99
weighting_scheme ="BISQUARE"
out_global_tbl = r"Outputs.gdb\Global_School_Colocation"
arcpy.stats.ColocationAnalysis(intype, infc_interest, outfc, field_interest,
time_field, cat_interest, infc_neigh, field_neigh,
time_field_neigh, cat_neigh, neighborhood_type,
num_neighbors, dist_band, swm_file, temporal_type,
time_step_interval num_permutation, weighting_scheme,
out_global_tbl)
# Categories from different datasets without categories
# Find the colocation of schools and hospitals
intype = "DATASETS_WITHOUT_CATEGORIES"
infc_interest = r"Colocation.gdb\Schools"
outfc = r"Outputs.gdb\Schools_Hospitals"
field_interest = ""
time_field = ""
cat_interest = ""
infc_neigh = r"Colocation.gdb\Hospitals"
field_neigh = ""
time_field_neigh = ""
cat_neigh = ""
neighborhood_type = "DISTANCE_BAND"
num_neighbors = ""
dist_band = "30 Kilometers"
swm_file = ""
temporal_type = ""
time_step_interval = ""
num_permutation = 199
weighting_scheme ="GAUSSIAN"
out_global_tbl = ""
arcpy.stats.ColocationAnalysis(intype, infc_interest, outfc, field_interest,
time_field, cat_interest, infc_neigh, field_neigh,
time_field_neigh, cat_neigh, neighborhood_type,
num_neighbors, dist_band, swm_file, temporal_type,
time_step_interval num_permutation, weighting_scheme,
out_global_tbl)
# Categories from two datasets
# Find the colocation of elementary schools and hospitals
intype = "TWO_DATASETS"
infc_interest = r"Colocation.gdb\Schools"
outfc = r"Outputs.gdb\Elementary_Hospitals"
field_interest = "Facility_Type"
time_field = ""
cat_interest = "Elementary"
infc_neigh = r"Colocation.gdb\Hospitals"
field_neigh = ""
time_field_neigh = ""
cat_neigh = ""
neighborhood_type = "K_NEAREST_NEIGHBORS"
num_neighbors = 15
dist_band = ""
swm_file = ""
temporal_type = ""
time_step_interval = ""
num_permutation = 499
weighting_scheme ="NONE"
out_global_tbl = ""
arcpy.stats.ColocationAnalysis(intype, infc_interest, outfc, field_interest,
time_field, cat_interest, infc_neigh, field_neigh,
time_field_neigh, cat_neigh, neighborhood_type,
num_neighbors, dist_band, swm_file, temporal_type,
time_step_interval num_permutation, weighting_scheme,
out_global_tbl)
许可信息
- Basic: 是
- Standard: 是
- Advanced: 是